Compositional Systems Biology
Compositional Systems Biology is our software-driven approach to building a flexible infrastructure for integrative biological modeling. At its core is a generalizable architecture for composing multiscale simulations—enabling plug-and-play models, transparent data flow, and seamless integration across biological domains.
Our infrastructure development focuses on:
- Composition Framework: A standard for connecting heterogeneous processes using modular interfaces and orchestration patterns.
- Composition Schema: A unified format for defining models, data structures, and process interactions to enable reproducibility and automated composition.
- Compositional Software: Open-source tools supporting simulation runtime, schema validation, model annotation, and API-based deployment for cloud and local use.
- Biological Interface Definitions: Standard schemas for defining cells, molecules, and environments, ensuring consistency and extensibility across simulations.
- Collaborative Modeling Infrastructure: Enabling scientific collectives to register and reuse modular components across research domains.
Our goal is to reduce the friction of building and sharing complex biological models, creating an open ecosystem where multiscale simulations can evolve continuously with contributions from diverse research teams.
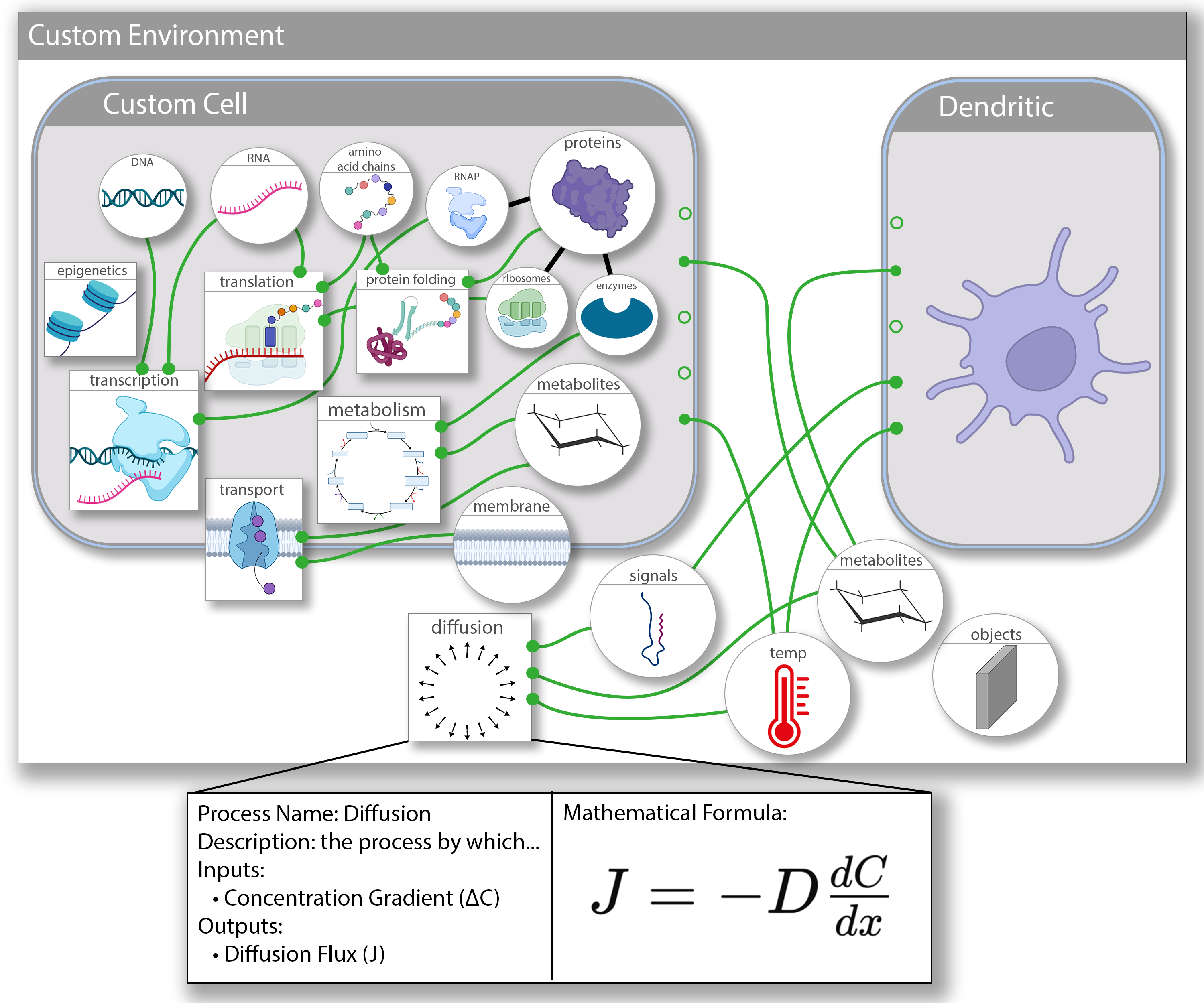
E. coli Whole-cell Model
We are developing a comprehensive, mechanistic model of E. coli that integrates gene regulation, metabolism, and cellular physiology to simulate behavior across molecular, cellular, and population scales. With over 19,000 parameters, it predicts gene expression, metabolite levels, protein interactions, and whole-cell properties such as biomass and growth rate—advancing our understanding of microbial systems biology.
Our focus is on using this model as a discovery tool to:
- Complete functional annotations by predicting roles of uncharacterized genes through simulation-data comparisons.
- Capture population heterogeneity by simulating variation in growth and physiology across thousands of cells.
- Model environmental context by exploring how nutrient conditions and stressors influence adaptation and behavior.
- Enable simulation-based inference using probabilistic and ML techniques for parameter estimation and experimental design.
By unifying diverse biological data in an extensible simulation, this work deepens our understanding of microbial physiology and enables systems-level reasoning and discovery.
Ocean Microbiome
Our research in ocean microbiomes is centered on connecting genome-scale metabolic models of bacteria and plankton to biogeochemical models of the water column. This integration aims to quantify the impact of microbial activities on climate. Key aspects include:
- Metabolic Modeling: Developing detailed models of microbial metabolism to understand the functional capabilities of ocean bacteria and plankton.
- Biogeochemical Integration: Linking these metabolic models to biogeochemical cycles in the water column to assess nutrient and carbon fluxes.
- Climate Impact: Quantifying how microbial processes influence global climate patterns through their roles in carbon sequestration and nutrient cycling.
This work is conducted in collaboration with the Center for Chemical Currencies of a Microbial Planet at the Woods Hole Oceanographic Institution.
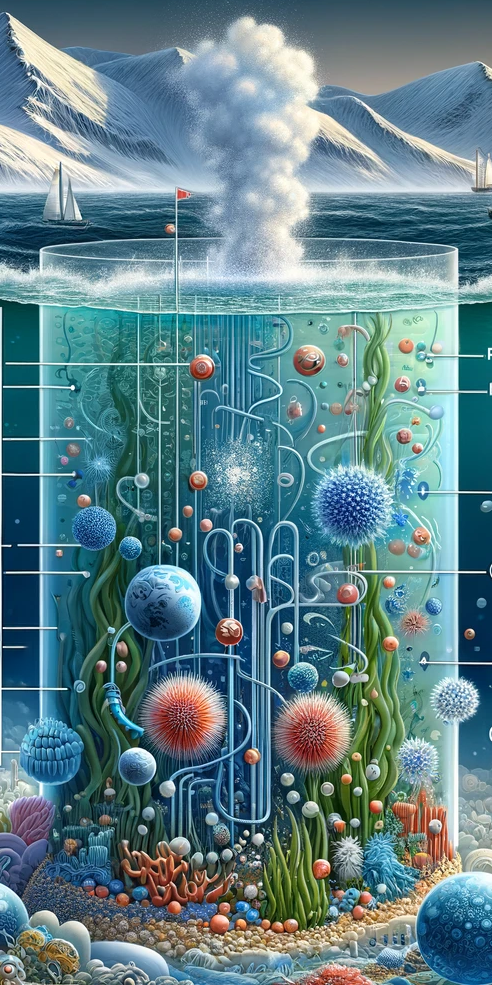
Gut Microbiome
Our gut microbiome research, conducted by UConn PhD students and undergrads in the Agmon lab, focuses on creating a comprehensive spatial dynamical model of the human intestines. Research areas include:
- Ecological Niches: Quantifying the various niches within the intestines and their unique microbial communities, and the spatially structured environment with flows driven by peristalsis.
- Microbial Community Interactions: Exploring how different microbes interact and coexist, from molecular networks to whole-gut physiology, including interactions between microbes and host cells, such as mucus production by goblet cells.
- Layered Complexity: Addressing the hierarchical complexity and diverse interactions within the gut microbiome.
- Dynamic Modeling: Beginning with stoichiometric modeling, adding multi-species interactions, and incorporating processes like digestion, absorption, and peristalsis.
- Multi-Omics Data Integration: Incorporating multi-omics data to build detailed spatial models of the gut environment.
Our goal is to integrate diverse datasets and uncover the complex microbial interactions that influence gut health and disease.
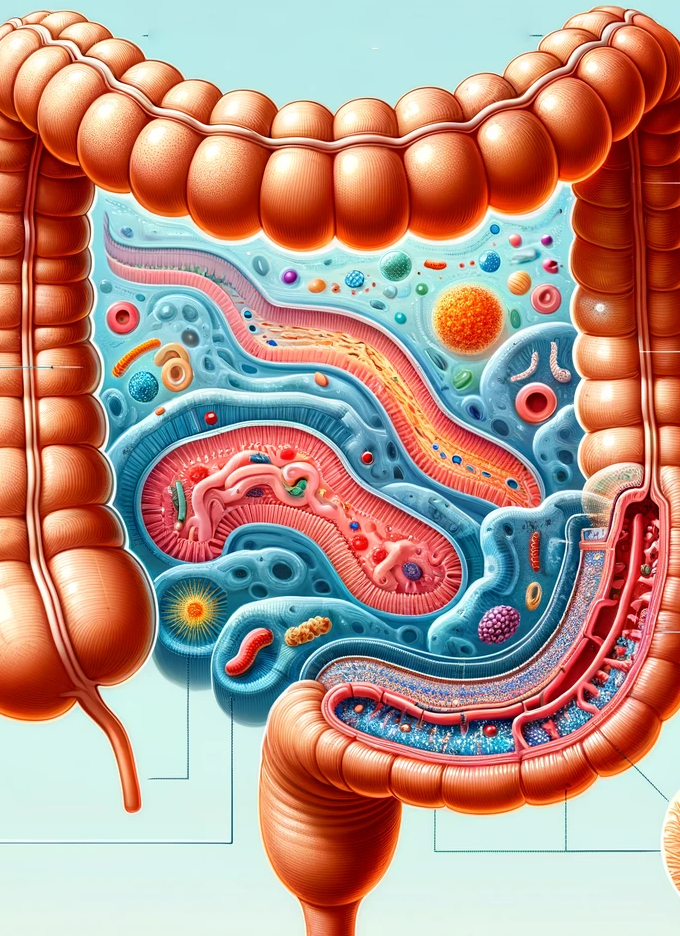